Unsupervised Person Image Generation with Semantic Parsing Transformation
Abstract
In this paper, we address unsupervised pose-guided person image generation, which is known challenging due to non-rigid deformation. Unlike previous methods learning a rock-hard direct mapping between human bodies, we propose a new pathway to decompose the hard mapping into two more accessible subtasks, namely, semantic parsing transformation and appearance generation. Firstly, a semantic generative network is proposed to transform between semantic parsing maps, in order to simplify the nonrigid deformation learning. Secondly, an appearance generative network learns to synthesize semantic-aware textures. Thirdly, we demonstrate that training our framework in an end-to-end manner further refines the semantic maps and final results accordingly. Our method is generalizable to other semantic-aware person image generation tasks, e.g., clothing texture transfer and controlled image manipulation. Experimental results demonstrate the superiority of our method on DeepFashion and Market-1501 datasets, especially in keeping the clothing attributes and better body shapes.
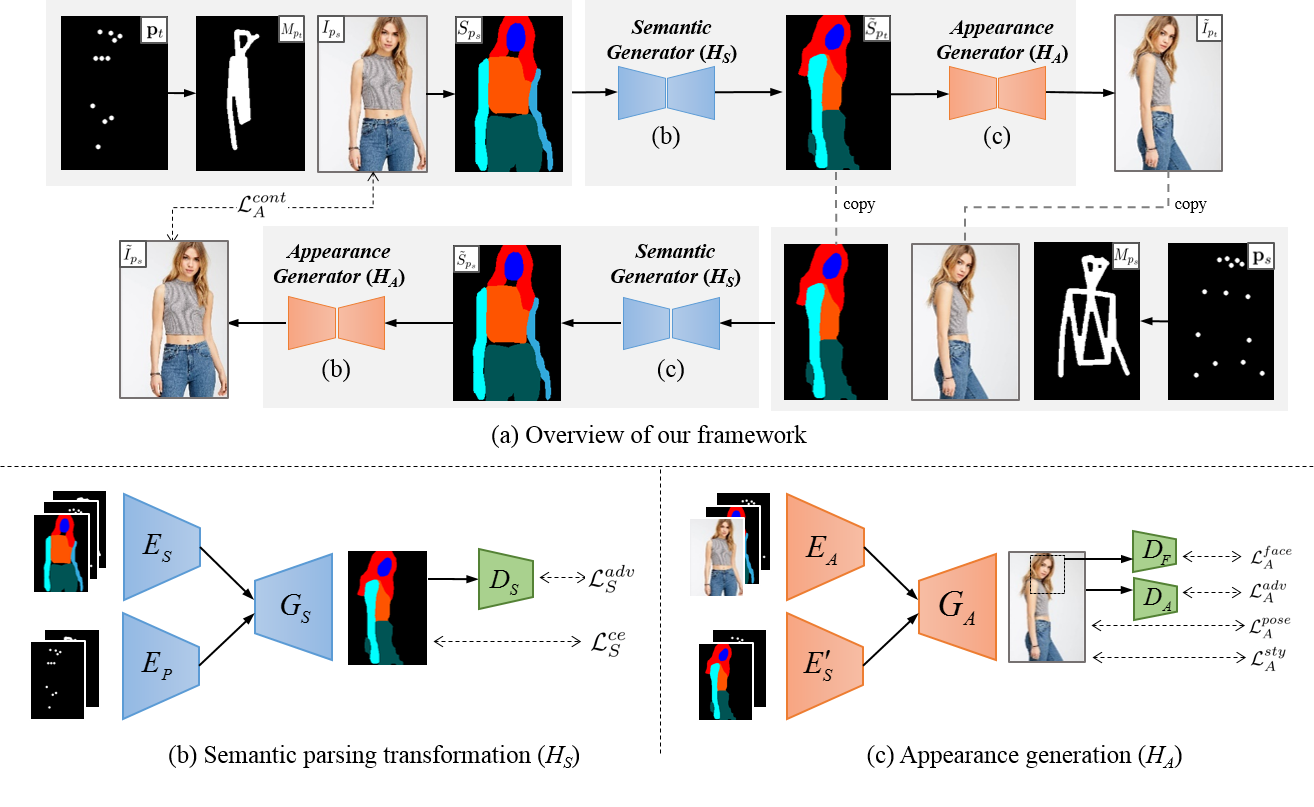
Fig.2 The framework of our model.
Results of Pose-Guided Image Generation
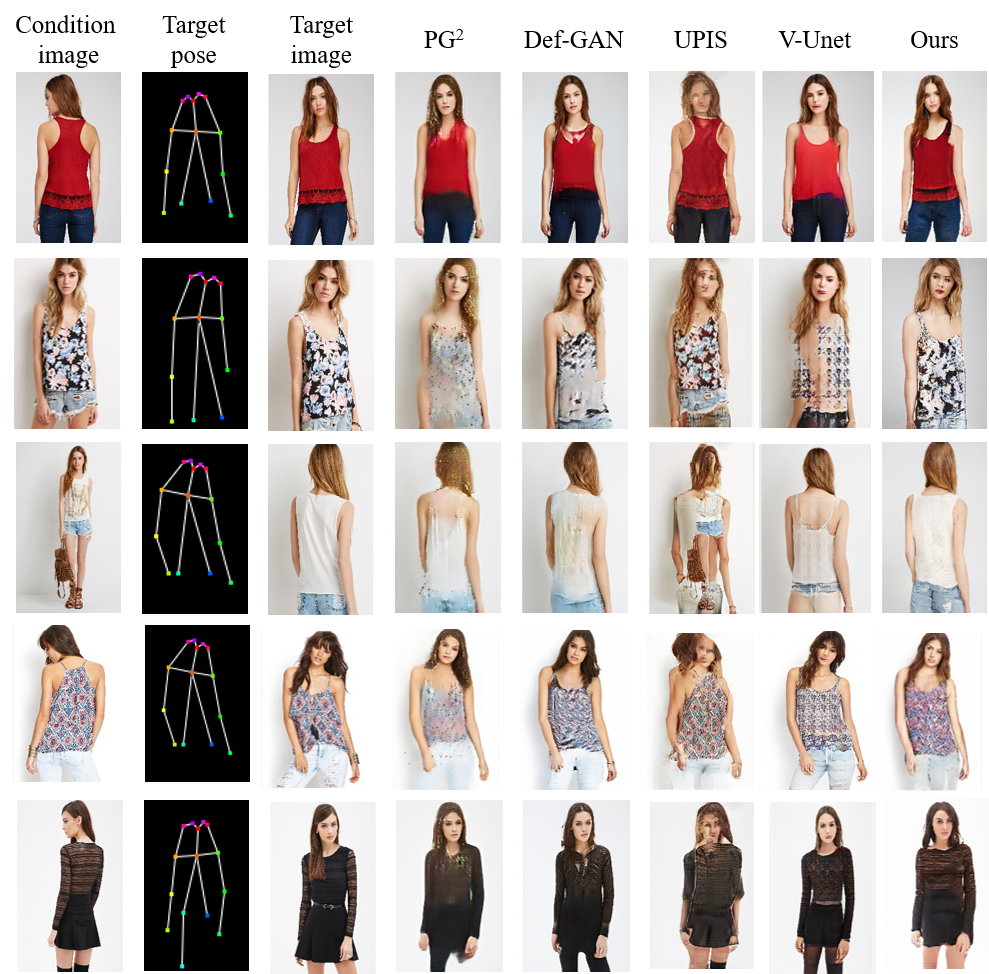
Fig.3 Visual results of different methods on DeepFashion. Compared with PG2[1], Def-GAN[2], UPIS[3], and V-Unet[4]. Our method successfully keeps the clothing attributes (e.g., textures) and generates better body shapes (e.g., arms).
Results of Clothing Texture Transfer
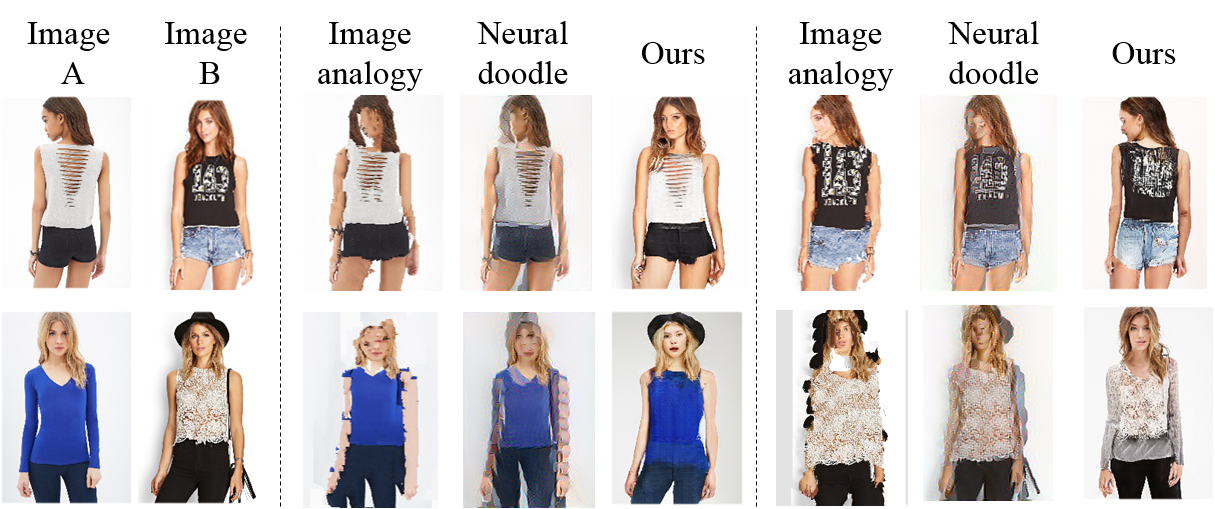
Fig.4 Application for clothing texture transfer. Left: condition and target images. Middle: transfer from A to B. Right: transfer from B to A. We compare our methods with image analogy[5] and neural doodle[6].
Results of Controlled Image Manipulation
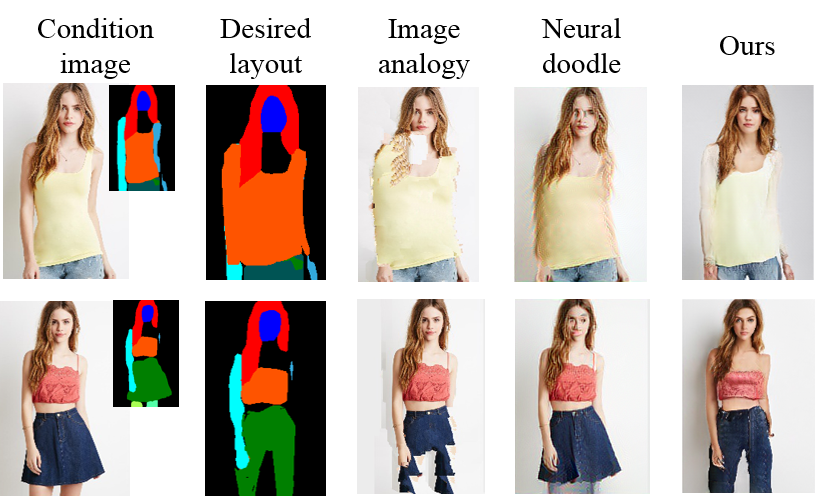
Fig.5 Application for controlled image manipulation. By manually modifying the semantic maps, we can control the image generation in the desired layout.
Reference
[1]. L. Ma, X. Jia, Q. Sun, B. Schiele, T. Tuytelaars, and L. Van Gool. Pose guided person image generation. In NIPS, 2017.
[2]. A. Siarohin, E. Sangineto, S. Lathuili`ere, and N. Sebe. Deformable gans for pose-based human image generation. In CVPR, 2018.
[3]. A. Pumarola, A. Agudo, A. Sanfeliu, and F. Moreno-Noguer. Unsupervised person image synthesis in arbitrary poses. In CVPR, 2018.
[4]. P. Esser, E. Sutter, and B. Ommer. A variational u-net for conditional appearance and shape generation. In CVPR, 2018.
[5]. A. Hertzmann. Image analogies. Proc Siggraph, 2001.
[6]. A. J. Champandard. Semantic style transfer and turning two-bit doodles into fine artworks. arXiv preprint arXiv: 1603.01768, 2016.